Build Effective Social and Behavior Change Strategies
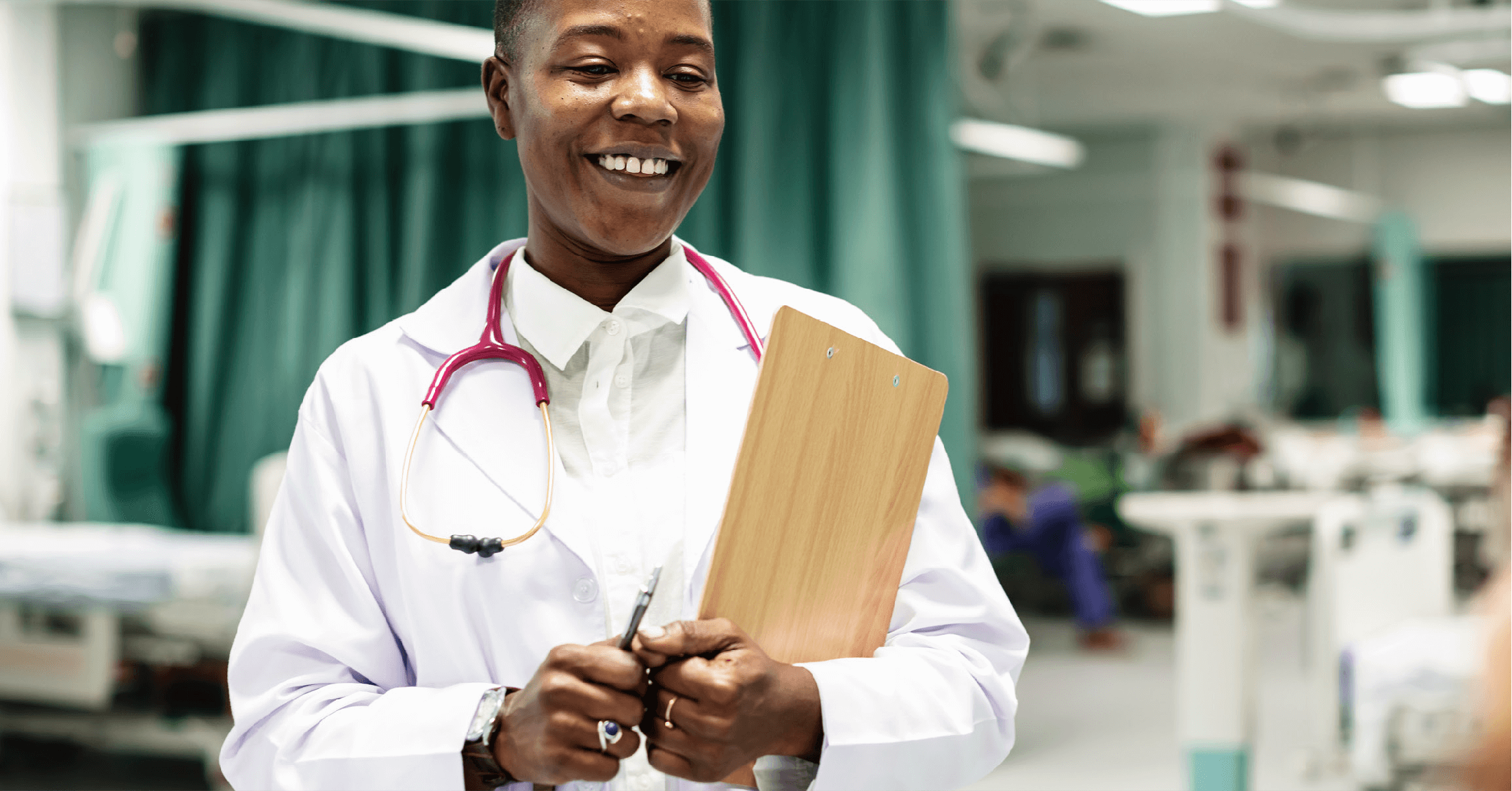
Big Picture
As world efforts to address infectious disease have made great strides in many parts of the developing world, countries in Sub-Saharan Africa continue to experience outbreaks of preventable disease. Between 2000 and 2015, 83% of cholera deaths reported to the World Health Organization (WHO) occurred in sub-Saharan Africa, a figure resulting both from high-mortality epidemics and persistent, endemic incidences. Cholera’s deadliness on the continent reflects a severe lack of access to improved water and sanitation infrastructure, and flooding quickly compounds the risk of disease.
This past month, Angola experienced the worst flooding in over a decade. The floods affected a country already at risk for cholera, with over half of the Angolans (56%, according to Fraym data) relying on unimproved drinking water and unfinished toilets. In light of this situation, Fraym’s hyper-local analysis that reveals concentration and characteristics of population at risk will support more targeted and effective prevention and surveillance strategies.
Outbreak Zone Analysis
Using Fraym data, we identified Cuanza Sul as one of the most vulnerable provinces to cholera based on access to water and sanitation indicators, among others. Also heavily affected in the 2006 cholera outbreak, only about 10 percent of the population in Cuanza Sul has access to improved drinking water and finished toilets. Next, we created a heatmap that pin-points concentrations of at-risk populations using machine learning. Although population is highly concentrated in cities, such as Sumbe, a significant number of at-risk people live in rural areas, particularly in the northwest part of the province.
Figure 1: Populations vulnerable to cholera are concentrated in rural areas of Cuanza Sul, where 90% lack access to improved water sources and finished toilet.
Intervention Planning
Drawing from its comprehensive library on population characteristics, Fraym produced a detailed portrait of the people living in Cuanza Sul to identify factors that differentiate at-risk communities. From this closer examination, Fraym data revealed several distinguishing features of vulnerable populations and their communities. In this region of Angola, vulnerable people receive less education, have limited media access, and are less likely to adopt hygiene practices, such as water treatment and handwashing.
Our findings have the potential to target and tailor cholera prevention efforts in the province, helping health workers reach and understand the communities who need it most. For example, with a notably higher illiteracy rate (48% among at-risk households compared to 26% among non-vulnerable ones), health education campaigns on cholera and prevention in Cuanza Sul’s vulnerable areas should be accessible and direct. Here, communities are not consuming media and have low literacy ability, meaning traditional mass media campaigns may have limited impact. Equipped with this information, implementing partners may consider community-based or non-traditional approaches to prepare these communities for future cholera outbreaks. A data-driven understanding of vulnerability to cholera and its contributing factors highlights this strategy and other avenues for impact.
Artificial intelligence and machine learning uncover new insights into people living with daunting challenges. In Angola, Fraym algorithms allowed our analysts to prioritize hot spots for deeper investigation and draw out an understanding of these populations from hundreds of data sources and indicators. This information positions health workers with a highly localized understanding of who these vulnerable people are, and the best means with which to reach them. For cholera and other infectious diseases, AI/ML can enable us to decrease preventable deaths in sub-Saharan Africa and across the developing world.