Build Effective Social and Behavior Change Strategies
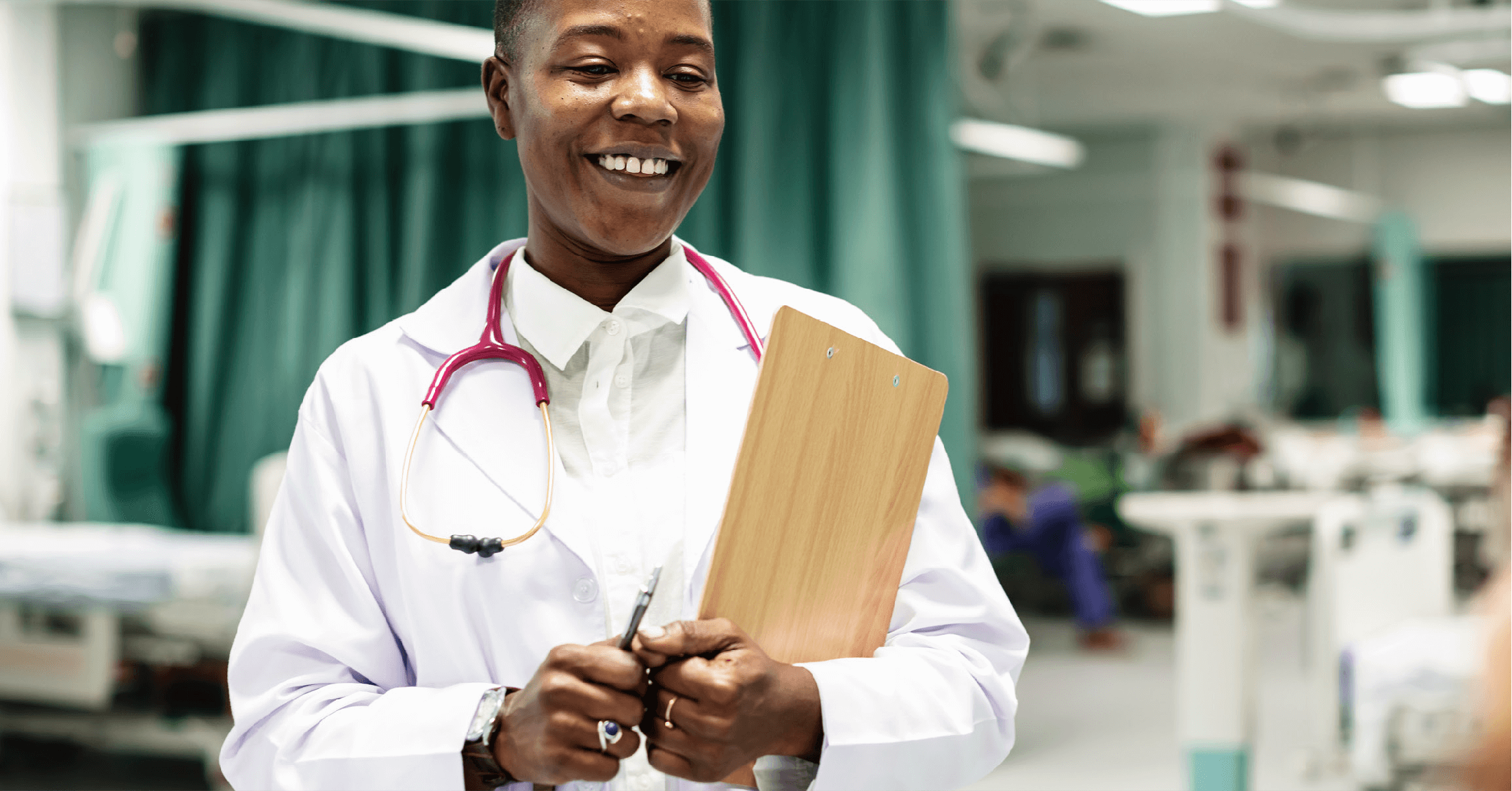
As countries around the world race to prevent and mitigate the growing threat of coronavirus, data on populations, their health characteristics, livelihoods, and location may prove to be invaluable for response efforts to a disease with significant unknowns. Advanced machine learning (ML) algorithms readily adapt and update available data to accommodate new learning, evolving risk factors, and newly exposed geographies as health officials learn more about this virus. Fraym recognized our unique position as a leader in this space, and we’ve begun to apply our local population data to rapidly enhance our understanding as the crisis evolves.
Understanding the spatial dynamics of at-risk populations can be important for health officials to guide response efforts like health infrastructure improvements, emergency funding allocation, preventative measures, and others. With this in mind, Fraym developed a risk profile of the population most at-risk to severe complications if they contract COVID-19 based on some of the early reporting from the most affected countries. We applied the model to Nigeria where health officials continue to announce new cases despite a strong early federal response.
We drew on three components that have been shown or expected to increase the risk of respiratory disease: people over age 60, regular smokers, and those who use dirty cooking fuel in their houses. Each component reflects individuals who live in households with the above attributes.
The result is a hyper-local heatmap of people most highly at-risk for life-threatening complications of COVID-19. In Nigeria, Fraym found that the LGAs of Ushongo, Vandeikya, and Konshisha in Benue state had one of the highest percentages of at-risk populations. Here, 11% of people, or around 100,000 residents, live in a household that fits our at-risk profile.
Communicating with at-risk regions is critical. Using neighborhood-level data on livelihoods and media consumption, public health officials can optimize campaigns based on medium and reach. For example, around 4 in 10 adults in these LGAs cannot read, which suggests that a radio campaign would be more effective in these areas.
For a disease impacting people at a global scale, hyper-local information on what may put people at risk, where to find concentrations of vulnerable populations, and the best means to reach them may radically transform the pandemic response. Fraym’s data repository and advanced ML algorithms can rapidly introduce data into a situation with significant unknowns and help inform a more data-led response that is targeted, efficient, and effective. As we recognize our responsibility and potential to transform decision-making during this challenging time, Fraym has already committed and is striving to make our data available to those combating the spread of coronavirus in Nigeria and around the world.