Build Effective Social and Behavior Change Strategies
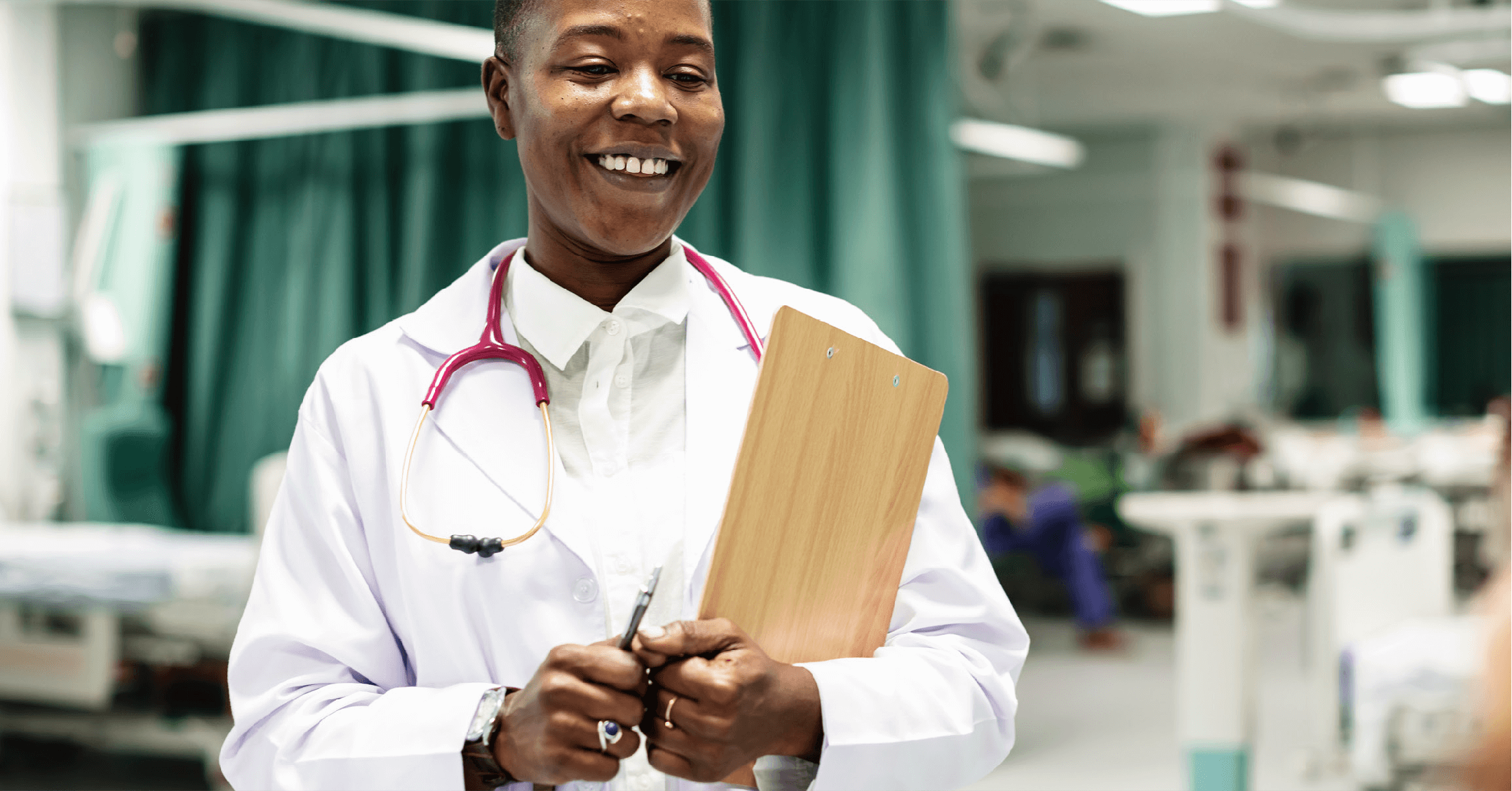
The operating environments of many development programs can be fluid, with unforeseen global and local challenges rising and falling over the course of a multi-year initiative. Within the past year alone, the emergence of COVID-19 has upended implementation strategies designed in a pre-pandemic world. Responsive MEL programming can reveal not just an activity’s impact but derive key insights to define a path forward. Swift and accessible dissemination of MEL findings are essential to ensuring that these insights are acted upon quickly, from high-level strategy to community-level reactiveness on the ground. Fraym’s machine learning (ML) algorithms and interactive platform were created to make large datasets and analysis actionable, not despite but especially in complex environments.
Fraym’s machine learning algorithms are designed to maximize existing datasets outside of their original functions. Fraym’s ML approach augments the usability of typical household-level data and reveals community-level trends that are relevant to implementers in the field. Paired with Fraym’s vast library of ML enhanced population information, any collected dataset benefits from a rich understanding of community-level livelihoods, vulnerabilities, and resources. Our high resolution heatmaps and geospatial analysis compile these otherwise disparate datasets into relevant and data-driven understanding of changing needs at the national or regional level, but also at the community level where strategy adjustments can have the greatest impact.
We make these learning accessible to those who need them most through our web-based platform, dataFraym®. Designed in response to growing demand for interactive, on-going access to Fraym data, dataFraym® combines both our ML enhanced datasets on hundreds of population indicators with our partners’ project data. Now, insights revealed from activity-specific MEL can be visualized alongside a breadth of contextual indicators, all at the community level. In Tanzania, partners at the World Bank used dataFraym® to locate and visualize communities that their project had so far failed to reach. By mapping their solar powered water pump locations over contextual maps such as pastoralist population, access to sanitation, and ability to pay, the team was able to quickly act upon these learnings and reach communities that had been missed before.