In collaboration with Facebook’s Project 17, Fraym explored the use of the Facebook Survey on Gender Equality at Home and high-resolution population data to unearth subnational differences in the male and female food security.
About the Project
Project 17 is Facebook’s partnerships-first approach to accelerating progress on the Sustainable Development Goals (SDGs). Its current area of focus is increasing the availability and use of gender data.
Our proof of concept largely focused on SDG 2: Zero Hunger, with a focus on the sex disaggregated responses to the question of “during the last 30 days, was there a time when you were worried about not having enough food because of lack of money or other resources?” in Nigeria. The data shows there was already a 7-percentage point difference in self-reported food security between men (79%) and women (86%). Were we to just apply this national average to the Facebook’s high resolution male and female population density maps, subnational differences would be constant at 7-percentage points. But again, our hypothesis was that this gender gap will likely differ by community based on the spatial differences we have seen in hundreds of subnational analyses.
Hyperlocal Targeting for Gender Disparity in Food Insecurity
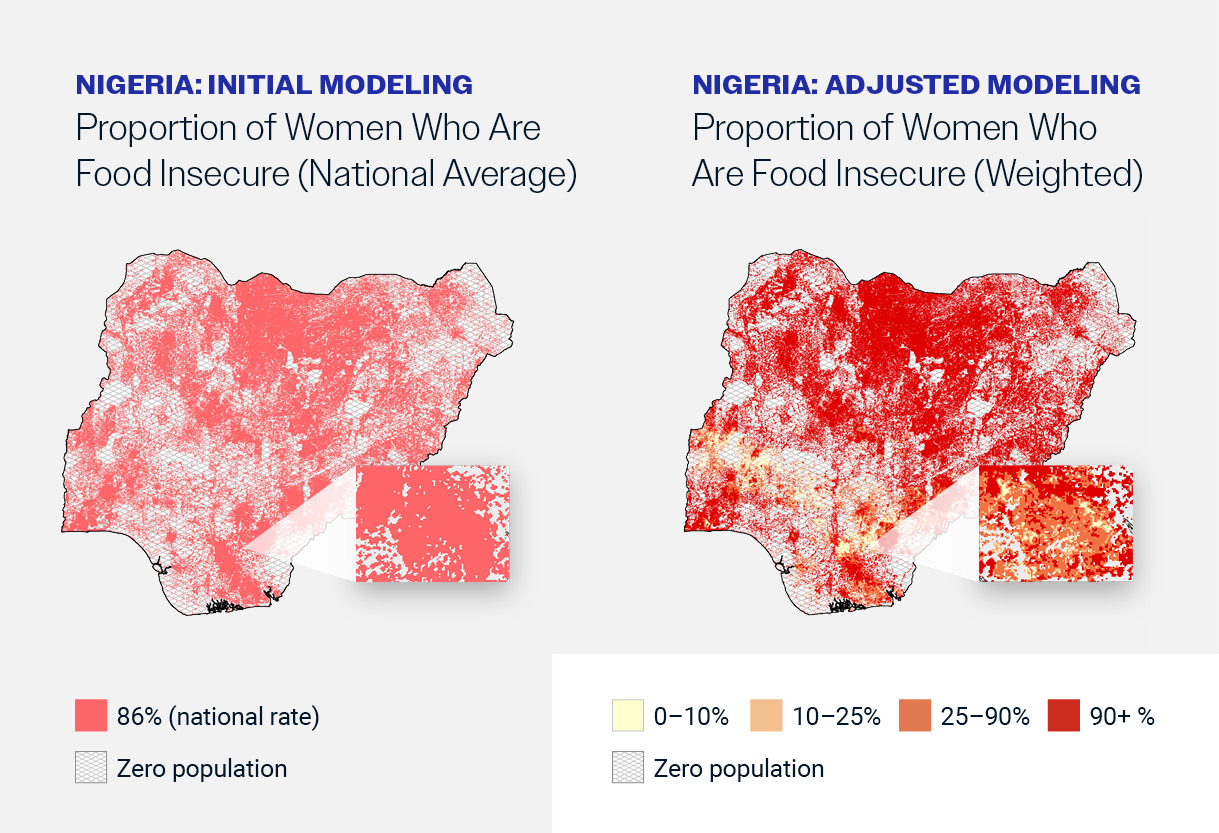
Our model indicates that there may be communities facing 25+ percentage point differences in food security representing enormous gender inequality in measuring SDG 2.
The result was a hotspot map of where the gender gap in food insecurity is very likely the largest.
This exercise identified that Imo, Rivers, Abia, Federal Capital Territory (FCT) and Plateau are states with the largest gender gaps in food security where women are more food insecure than men.
%
Of men reported food insecurity
%
Of women reported food insecurity
%+
Gender differences in hotspot communities
Understanding Spatial Differences Between Communities
In order to successfully measure the SDGs, we must take into account gender disparities by both generating more data and applying advanced analytical techniques to better understand them. At Fraym, we advocate for the use of hyperlocal data for decision-making because years of work has unearthed the stark spatial differences between communities.

“Facebook’s publicly available gender datasets are only meaningful when they are used to expose and address unseen gender gaps. By layering these insights with other data sources, Fraym has helped to reveal how this gender data can advance equality across the SDGs”