Who will bear the brunt of urban food shortages?
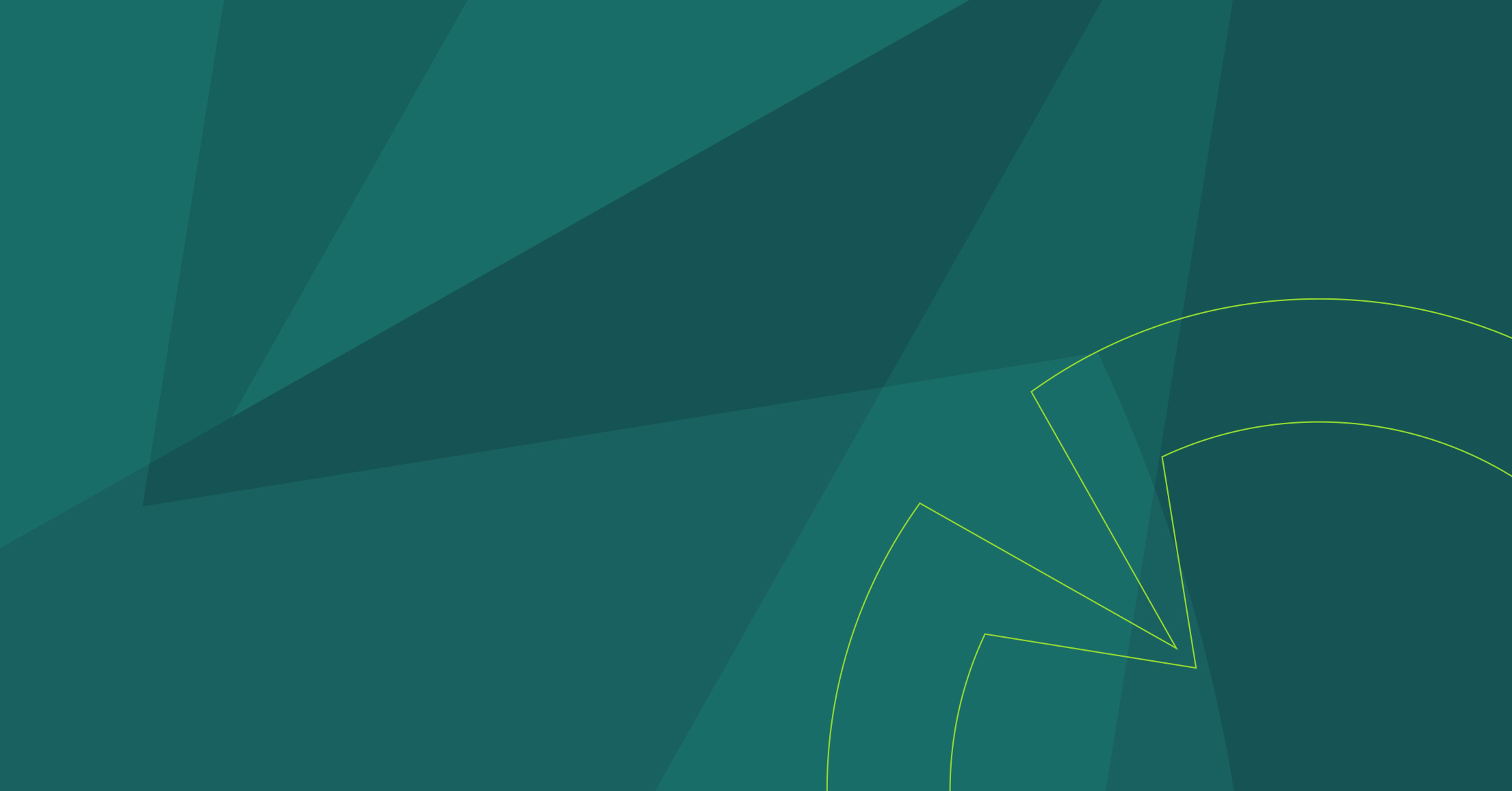
Achieving food security has always been a multifaceted and global challenge. However, the unprecedented consequences of conflict, climate, and COVID-19 on a global scale have only served to further increase and complicate that challenge.
Understanding local variation in food insecurity, both its current measures and its driving factors, is essential. Fraym data achieves this necessary level of granularity through existing survey methodologies and data collection efforts combined with cutting edge machine learning technology. As the issue of global hunger becomes more complex, a community-level understanding is needed to quantify and mitigate food insecurity and vulnerability to it.
The World Bank predicts that the Ukrainian conflict, including closure of Black Sea ports, will increase the number of people with severe hunger by 50 million people before the end of the year.
The International Food Policy Research Institute’s (IFPRI) 2022 Global Food Policy Report identified climate change as an additional major driver of food insecurity that researchers project will only continue to worsen, reducing agricultural yields, increasing prices, and adding to GHG emissions without necessary adaptation.
The COVID-19 pandemic continues to reverberate through the global economy, with already-vulnerable countries still grappling with high budget deficits, high unemployment, and rising inflation constraining governments’ abilities to counter domestic food price hikes.
To address immediate challenges, Fraym created heatmaps of food insecurity based on the IFPRI’s Global Hunger Index for targeting humanitarian responses. Currently, implementers rely on many publicly available initiatives to map, project, and respond to hunger needs, however, these traditional maps are limited to administrative boundaries that mask critical differences at the community-level.
In the longer term, Fraym’s data on attitudes, perceptions, and concerns reveal insights on specific populations. Two neighborhoods with similar measures of current food insecurity can attribute their situation to wildly different factors requiring distinct solutions.
For example, Fraym data on COVID-19’s disproportionate impact on women revealed clear spatial variation in how the pandemic affected their economic status beyond loss of income.
In India, over half of women entrepreneurs either lost jobs or had their working hours reduced, while over half of women nationally simultaneously saw increases in hours devoted to unpaid work in the home.
Subnationally, Chandigarth state tops the list for both concerns: 86% of women saw an increase in unpaid work due to COVID-19 in this state, while 57% of women entrepreneurs in Chandigarth saw reductions in paid hours. Both groups of women are at higher risk to food insecurity due to future income shocks – and nowhere higher than in Chandigarth.
With emerging and evolving challenges, solving global hunger is increasingly complicated. Fortunately, technology like Fraym’s ML generated data can match the sophistication of the problem and deliver actionable insights for decision makers.