Market Segmentation for Health Programs
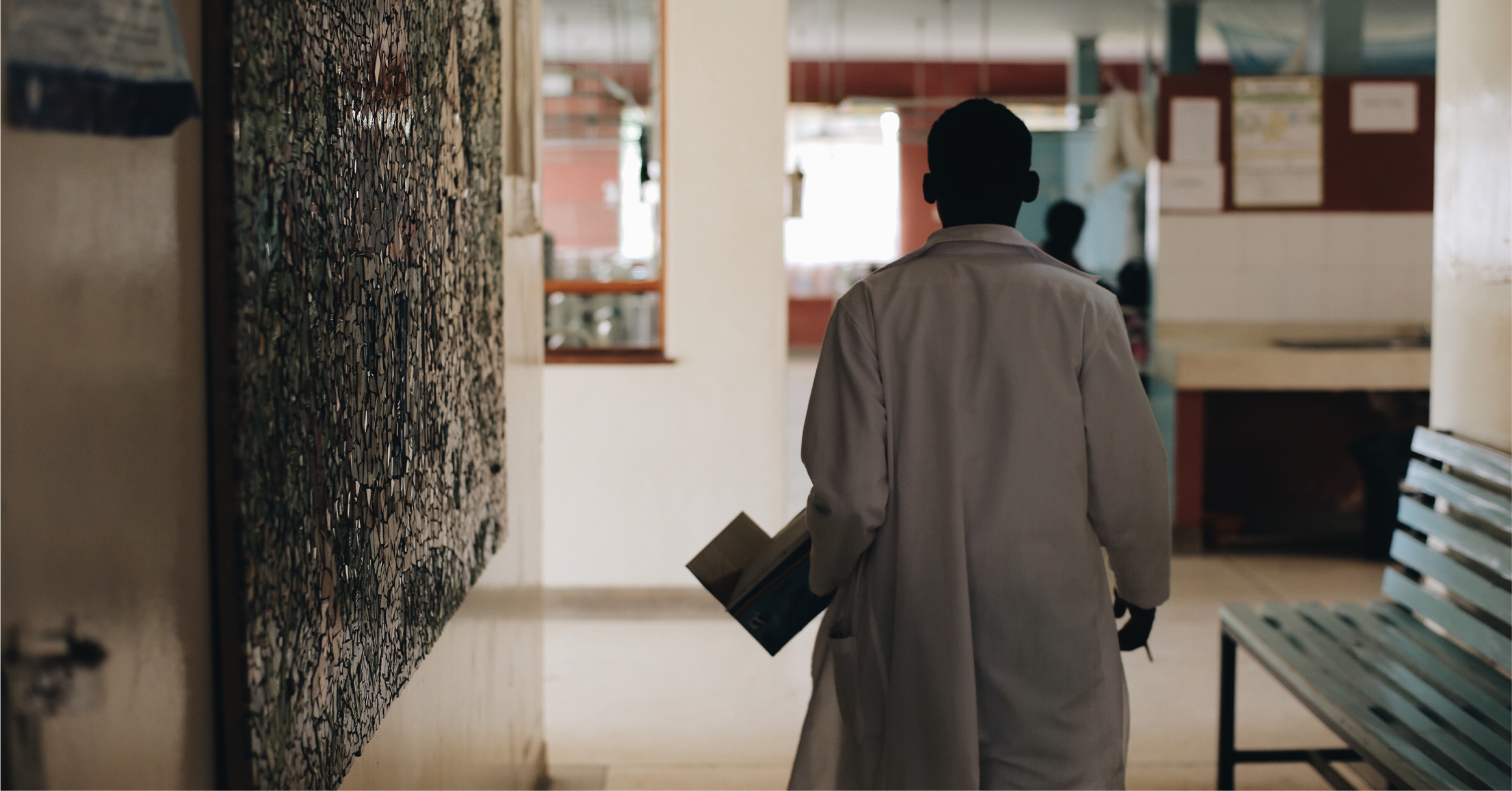
CASE STUDIES
The Challenge
Johnson & Johnson’s Global Public Health team partnered with Fraym to use cutting-edge technology to understand vaccine hesitancy in low- and middle-income countries. The Sabin Vaccine Institute published the research in BioMed Central (BMC Proceedings) as part of the Vaccination Acceptance Research Network’s 2022 Conference journal supplement (#VARN2022).
At the onset of the COVID-19 pandemic, teams working to distribute vaccines had virtually no data regarding the determinants of vaccine hesitancy. When data was available, it was often aggregated at the national level which masked critical local differences. This missing information was essential for public health stakeholders to tailor their interventions and increase vaccine uptake. For two-years, J&J and Fraym worked together to close this data gap in 10 countries.
Our groundbreaking collaboration showed, for the first time, that it’s possible to use machine learning to spatially interpolate attitudinal data at scale. To support local vaccine uptake, this study focused on estimating attitudinal data on the determinants of vaccine hesitancy as framed by the WHO’s Confidence, Complacency, and Convenience (“3Cs”) model.
In a recently published BMC Proceedings (BioMed Central) article, Fraym researchers describe the methods they used to reliably predict why people were hesitant to get the vaccine. Below are excerpts from the article.
Methods
To support local vaccine uptake, we focused on estimating attitudinal data on the determinants of vaccine hesitancy as framed by the WHO’s Confidence, Complacency, and Convenience (“3Cs”) Model of Vaccine Hesitancy. A nationally and regionally representative household survey of 4,922 adults aged 18 and above was collected in February 2022. This custom survey was designed to collect information on population demographics and socioeconomic characteristics, as well as attitudes towards COVID-19 and concerns about the COVID-19 vaccine. We developed Multiple Correspondence Analysis (MCA) indices of each of the 3Cs, all of which include attitudinal components. A machine learning (ML) framework was used to spatially interpolate these indices at a one square kilometer (1km2) resolution using approximately 130 spatial covariates from high-resolution satellite imagery, and 24 covariates from the 2018 Nigeria Demographic and Health Survey (DHS).
Mapping low confidence in the vaccine at the national level.
Here are the LGAs where a majority of the population are Facebook users, have access to a mobile phone, and live in a community that has a majority of people with low confidence in the COVID-19 vaccine.
If you want to run ads in one language, you can break it down to different regions of the country. Here’s an example of Housa speakers that fit the criteria above – a majority of the population is FB users, have mobile phone access, and live in a community that has majority low confidence in the COVID-19 vaccine.
%
Nigerians have low confidence in the vaccine
%
Nigerians on Facebook
%
of Nigerians have access to a smartphone
The findings demonstrate that:
1. Attitudinal population data, much like demographic and socioeconomic population data, can be reliably estimated and mapped at the hyperlocal level.
2. This attitudinal data on COVID-19 vaccination can be used to develop robust indices that measure the determinants of vaccine hesitancy at the hyperlocal level.
3. The drivers and barriers of COVID-19 vaccine uptake have large spatial variance that cannot be captured through national surveys and aggregate statistics alone.